AUV Technology
Annotated list with links to reports or papers.

Risk Management
Recent concepts, developments and practical experience
Dynamic risk analysis with an Antarctic under ice case study
Early risk analyses of AUV deployments under ice considered a fixed environment description, either as broad headings such as 'sea ice' or 'shelf ice', e.g. Brito et al. (2010) or provided a more detailed initial menu covering environment and capabilities, such as the Bayesian Belief Network approach in Griffiths and Brito (2008). Yang et al. (2020) have developed a new Bayesian approach that enables dynamic changes in the operating environment to affect the risk assessment. The dynamic Bayesian network incorporates information on the AUV's location, from which estimates of the environmental factors can be determined, and incorporated in the risk analysis. Furthermore, if there is a communications link between operators and the vehicle the mission path can be altered to reduce risk by avoiding those paths that would encounter hazards, for instance ice keels.
The authors showed how their method could be used in practice through using the open water, sea ice and ice shelf environments and risks encountered by the Autosub3 AUV on its Pine Island campaign in 2009. Conditional Probability Tables are presented for the operating environments and, importantly, also for prediction uncertainty, resulting in an example time history of the risk of damage.
A risk-averse approach to polar AUV mission planning
Despite AUV operations in Polar Regions being acknowledged as high risk King et al. (2020) show that a risk-averse approach to mission planning is feasible. Main elements of their approach include:
• Seek to refresh knowledge of best practices through open and frank discussion among subject matter experts including those with practical experience of AUV operations in polar environments.
• Set out mission by mission objectives starting with low risk missions before attempting those with higer risk.
• Progress to riskier missions only if prior missions successful and performance well understood.
• Define preliminary safe versions of riskier missions - e.g. carry out in open water.
• All major faults to result in a halt to mission risk progression.
• Recognise risk in launch and recovery operations - leave a working AUV in the water as long as feasible.
This approach worked well for the deployments of the nupiri muka AUV in the vicinity of the Sørsdal Glacier in 2018 and the Thwaites Glacier in 2019–20.
A Bayesian approach to real-time control of an AUV applied to under ice operations
The Bayesian approach adopted by Brito and Griffiths (2016) addressed the question of predicting risk of loss prior to undertaking an AUV mission. Bremnes et al. (2020) propose embedding Bayesian principles within the supervisory control algorithms in a vehicle. Such an approach embeds risk management within the software handling sub-system functions such as the guidance law for following the underside of sea or shelf ice utilising sonar distance estimates and pitch and depth control. The concept of an overall Bayesian network covering multiple vehicle sub-systems to provide a holistic assessment of risk at any instant is also explored through simulations.
Including human factors in risk analysis for AUV operations in the Antarctic
There is now a substantial body of literature on AUV risk analysis for operations in the Polar Regions based on the technical aspects of sub-systems, deployment and recovery, support vessel characteristics and the operating environment. However, organisational and human factors have, to the most part, been neglected. Loh et al. (2019) address these aspects of risk analysis - factors that can be difficult to quantify, or even ascertain due to reticence or reluctance to disclose problems, including, at times, deep institutional root causes.
Recognising the challenges in quantifying these factors, they propose a hybrid fuzzy system dynamics risk analysis (FuSDRA). In this approach the system dynamics elements capture those parts that are time dependent, e.g. growth in experience of an established and coherent team, with the fuzzy logic recognises the uncertainty. A comprehensive causal structure map applicable to estimating loss of the AUV nupiri muka in the Antarctic included four sub-models for: Budget; Utilisation; Technical Reliability and Human Reliability (see their Figure 8).
Meta analysis of AUV risk analysis papers
Chen et al. (2021) analysed critical risk factors and causal relationships in AUV operations as described in 42 published papers. The authors also documented and commented on how the topic of AUV risk and reliability analysis has evolved over the last 20 years. The categorised methods as qualitative, semi-quantitative and quantitative, with work over the last 10 years focussing on quantitative methods. Increased effort on dynamic risk analysis, addressing limited historical data, intelligent and multi-vehicle risk analysis are key topics for future research.
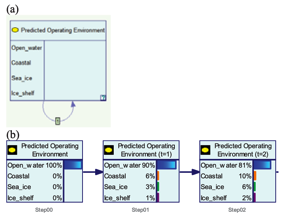
From Yang et al. (2020), an extract from the Dynamic Bayesian Network able to estimate risk in multiple operating environments.
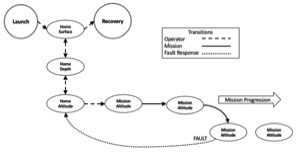
State transitions diagram for a risk-averse fault-terminated mission near the Thwaites Glacier for the AUV nupiri muka in 2019–20, from King et al. (2020).
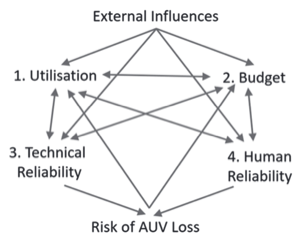
Outline of the causal loop diagram from Loh et al. (2019) that includes human and organisational factors alongside technical reliability together with their interacting feedback loops.
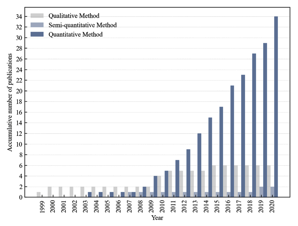
A cumulative frequency graph from Chen et al. (2021) showing increase in the number of papers on AUV reliability, and a move from qualitative to quantitative methods.